GSS/SSS/ASA PD Virtual Workshops on “The World of Blended Data” a Big Success –
Practicum including Student Showcase this Fall
Elizabeth Mannshardt and Jenny Thompson
The Government Statistics Section (GSS) and Social Statistics Section (SSS) hosted a series of virtual workshops, offered as a part of the ASA Professional Development Program. This free series targeted audiences who may not be able to travel to conferences but are interested in continuing education opportunities. Each virtual workshop consisted of a 1-hour presentation followed by a virtual participation in a group discussion and activities using data and code provided by the presenter. The six sessions had between 75 and 120 attendees each. Due to the overwhelming success of the Virtual Workshop, GSS and SSS are excited to announce the series will continue in Fall 2020 via a Virtual Workshop Practicum including a Student Showcase, hosted again as part of the ASA’s Professional Development Series. Practicum sessions will focus on user applications – both completed and in progress—from a variety of methodologists ranging from current students to seasoned professionals. The Virtual Workshop and corresponding Practicum framework are particularly timely given the current travel and conference limitations. Virtual Workshop participant feedback and suggestions for future offerings are presented here along with details for the Fall Practicum.
The original Virtual Workshop series exposed participants to the advantages of using combined data sources for developing inferential models and measures, while remaining cognizant of the challenges associated with combining large datasets and the potential pitfalls of analyses of blended data, including privacy considerations. Topics covered included an Overview on Blended Data (Frauke Kreuter, University of Maryland); Intro to Big Data and ML for Survey Researchers (Trent Buskirk, Bowling Green State University); How Rare is Rare? The Importance of Validation (Aric LaBarr, North Carolina State University); Intro to Python for Data Science (Hunter Glanz, California Polytechnic State University); Interpretability vs. Explainability in ML for High Stakes Decisions (Cynthia Rudin, Duke University); and Differential Privacy (Matthew Graham, US Census). All webinar materials and videos are available on the GSS Professional Development and Mentoring website.
After the final workshop in the series, ASA Professional Development conducted a survey of workshop participants. Although the response pool was small (32 participants), feedback was overwhelmingly positive, with participants providing very useful suggestions on both topics and logistics for possible future offerings. Overall, the Virtual Workshop earned 4.4/5 rating from 32 respondents. The evaluation provided some evidence of the relevance of the suite of selected topics, with the majority of respondents agreeing that “The concepts presented will inform my practice” (25/32) and that “The tools highlighted will be useful in my practice” (25/32).
The benefits of virtual presentations to a broad audience were underscored, with the workshop’s most valuable aspects being “community engagement” and “Being able to learn without travel”: one participant commented “Not being based in North America it gave me the opportunity to hear from experts I would not get to hear” Following a rating of “Strongly Agree” that the tools will be useful in practice, a participant stated why: “The distance learning aspect - hands down. It made this workshop very accessible to audiences!”
Participant feedback also included: “Very relevant topics, good speakers”, “Lots of new and interesting information, well-presented”, and “These types of workshops are a real benefit”. The Virtual Workshop offered multiple introductory topics – “Excellent introduction”, “this workshop was a great introduction”, and “this was a very helpful jump-start to facilitate my own analyses”. A participant attending five of the six sessions commented, “The lectures were informative, and the lecturers were entertaining and knowledgeable”, with an attendee of four sessions stating, “Very educational and at my level”.
Suggestions for going forward included an open discussion board among attendees to be used during and after the webinar; additional readings and examples for further study and practice; and deeper-dive multi-part tutorials on certain topics. Several participants also expressed interest in more sessions on various topics – stay tuned!
In Fall 2020 GSS, SSS, and ASA PD will offer a Virtual Workshop Practicum. Virtual Workshop organizers will share our experiences putting the techniques and lessons learned into practice in a virtual setting with a large statistical community. Sessions focusing on user applications will highlight work from career professionals and seasoned practitioners in the area of Blended Data. In addition, Practicum offerings will include a Student Showcase. Students often have limited travel funds, and given current events travel is an uncertainty for all. This Student Showcase will provide a great way for students to highlight their work to the statistics community via this highly attended Virtual Workshop. Students and professionals are invited to submit their projects putting blended data techniques into practice for the opportunity to present in the upcoming Virtual Workshop Practicum. Full details on the GSS Virtual Workshop Practicum site.
2019/2020 Virtual Workshop Materials are now on GitHub! https://github.com/zzlalo/2019-2020GSSVirtualWorkshops
Topics, Presenters, and Materials:
Overview by Frauke Kreuter, Director of Joint Program in Survey Methodology, University of Maryland
(slides) (Video accessible via Dropbox)
- Broad introduction to subject, showing how correctly blended data can lead to enhanced inference.
- Emphasis on applications in surveys and censuses.
- Discussions of challenges and some pitfalls/dangers of analyses on blended data including privacy considerations
|
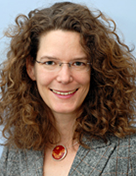 |
How Rare is Rare? The Importance of Validation, Professor Aric LaBarr, North Carolina State University's Institute for Advanced Analytics.
(slides) (R code) (Video accesible via Dropbox)
Abstract: In the growing world of data science and analytics, data is becoming more prevalent and used by organizations from every field. However, we must be careful that the pendulum doesn't swing too far to one side and we start torturing data into saying whatever we want. Much like in clinical trials where we have placebos, we need to properly validate our results and models to better understand if we have a "placebo effect" in our model results and we aren't just getting lucky. This talk highlights the technique of target shuffling, which tries to answer that exact question - what is the probability that my results occurred due to random chance? First used in hedge fund strategy validation, this simulation based technique answers this question for any cross-sectional data problem in an easily interpretable way.
Bio: Aric is passionate about helping people solve and analyze problems with their data. Aric is a faculty member at the Institute for Advanced Analytics, the nation's first master of science in analytics degree program. Aric helps innovate the current structure of education to better prepare a modern work force ready to communicate and handle a data driven future. He develops and teaches courses in statistics, mathematics, finance, risk management, and operations research. His focus is to teach, mentor, and serve students as well as businesses on modern analytic techniques for making data driven decisions.
Aric's NC ASA webinar "What Data Science Means to an Executive": https://youtu.be/Czko10HiNvc |
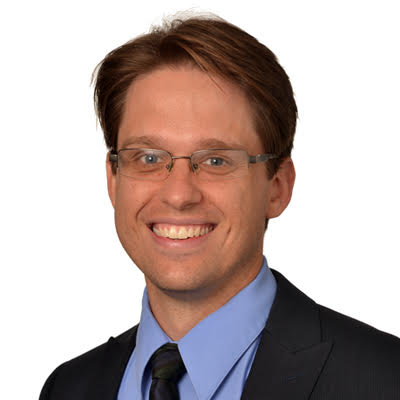 |
Interpretability vs. Explainability in Machine Learning for High Stakes Decisions, presented by Professor Cynthia Rudin, Duke University
(Video accesible via Dropbox)
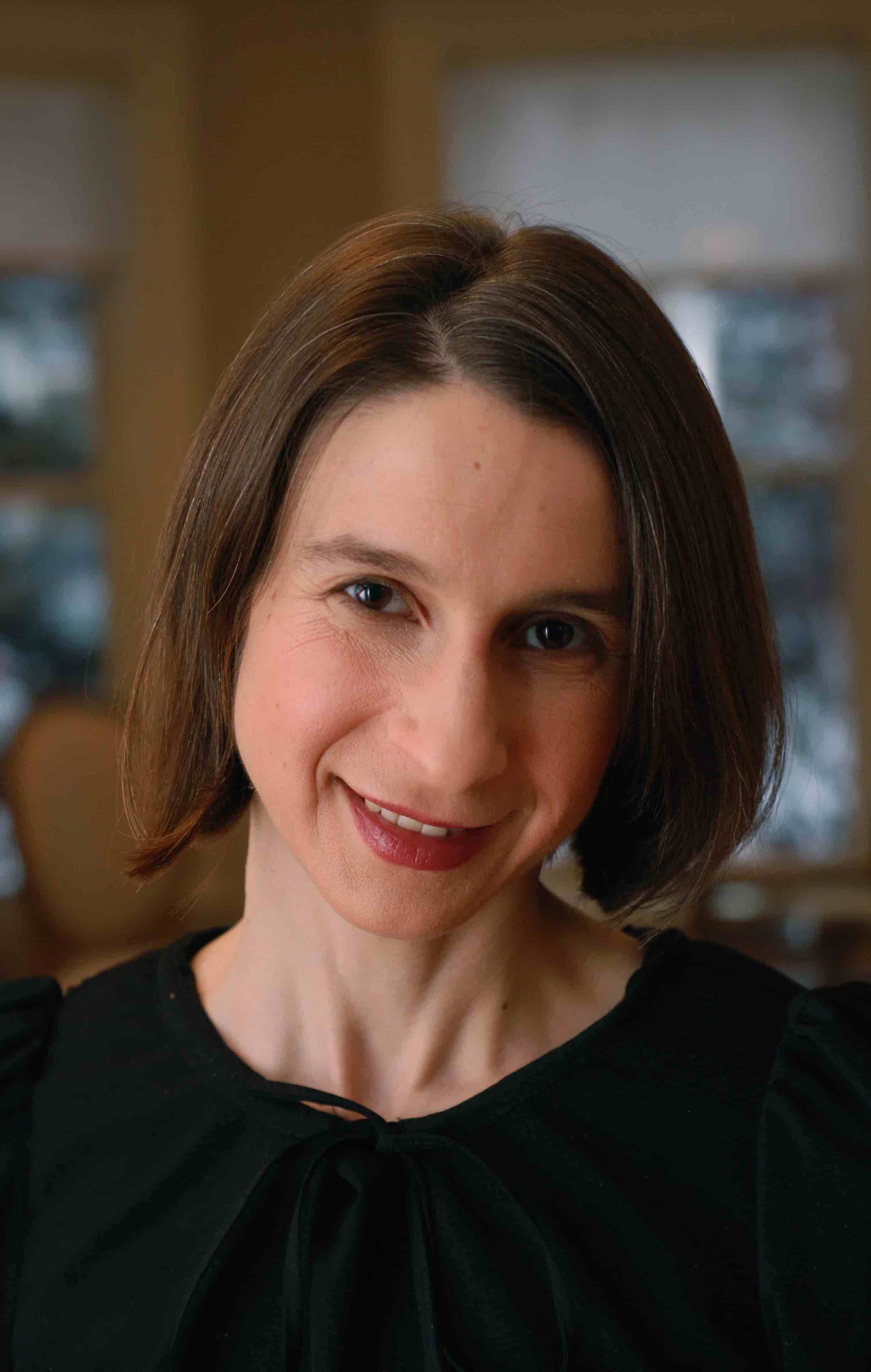 |
Abstract: With widespread use of machine learning, there have been serious societal consequences from using black box models for high-stakes decisions, including flawed bail and parole decisions in criminal justice. Explanations for black box models are not reliable, and can be misleading. If we use interpretable machine learning models, they come with their own explanations, which are faithful to what the model actually computes. In this talk, I will discuss some of the reasons that black boxes with explanations can go wrong, whereas using inherently interpretable models would not have these same problems. I will give an example of where an explanation of a black box model went wrong, in particular I will discuss ProPublica's analysis of the COMPAS model used in the criminal justice system: ProPublica’s explanation of the black box model COMPAS was flawed because it relied on wrong assumptions to identify the race variable as being important. Luckily in recidivism prediction applications, black box models are not needed because inherently interpretable models exist that are just as accurate as COMPAS. I will give examples of such interpretable models in criminal justice and also in healthcare.
Bio: Cynthia Rudin is an Associate Professor of computer science, electrical and computer engineering, and statistics at Duke University, and directs the Prediction Analysis Lab. Previously, Prof. Rudin held positions at MIT, Columbia, and NYU. She is the recipient of the 2013 and 2016 INFORMS Innovative Applications in Analytics Awards, an NSF CAREER award, was named as one of the "Top 40 Under 40" by Poets and Quants in 2015, and was named by Businessinsider.com as one of the 12 most impressive professors at MIT in 2015. Work from her lab has won 10 best paper awards.
Cynthia is past chair of the INFORMS Data Mining Section, and is currently chair of the Statistical Learning and Data Science section of the American Statistical Association. Her research focuses on machine learning tools that help humans make better decisions. This includes the design of algorithms for interpretable machine learning, interpretable policy design, variable importance measures, causal inference methods, new forms of decision theory, ranking methods that assist with prioritization, uncertainty quantification, and methods that can incorporate domain-based constraints and other types of domain knowledge into machine learning. These techniques are applied to critical societal problems in criminology, healthcare, and energy grid reliability.
|
Introduction to Big Data and Machine Learning for Survey Researchers, by Trent Buskirk, Novak Professor of Data Science, Bowling Green University
(materials)
|
|
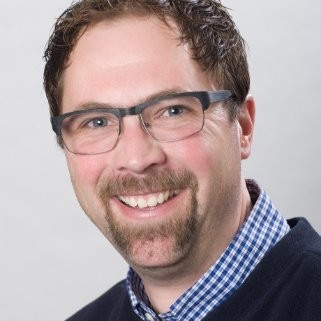 |
- Overview of Big Data terminology and concepts
- Introduction to common data generating processes
- Primary issues in linking Big Data to survey data
- Pitfalls in inference with Big Data
- Detailed applications/examples using R and Python
|
|
|
Introduction to Python for Data Science, by Hunter Glanz, Assistant Professor, Department of Statistics, California Polytechnic State University
(materials)
- Introduction to Python for data manipulation in preparation for machine learning.
- Examples using open source government data.
- Emphasis on data wrangling and exploratory data analysis including visualizations.
Bio: Hunter Glanz is an Assistant Professor of Statistics and Data Science at California Polytechnic State University (Cal Poly, San Luis Obispo). He received a BS in Mathematics and a BS in Statistics from Cal Poly, San Luis Obispo followed by an MA and PhD in Statistics from Boston University. He maintains a passion for machine learning and statistical computing, and enjoys advancing education efforts in these areas. In particular, Cal Poly’s courses in R, SAS, and Python give him the opportunity to connect students with exciting data science topics amidst a firm grounding in communication of statistical ideas. Hunter serves on numerous committees and organizations dedicated to delivering cutting edge statistical and data science content to students and professionals alike. In particular, the ASA’s DataFest event at UCLA has been an extremely rewarding experience for the teams of Cal Poly students Hunter has had the pleasure of advising.
|
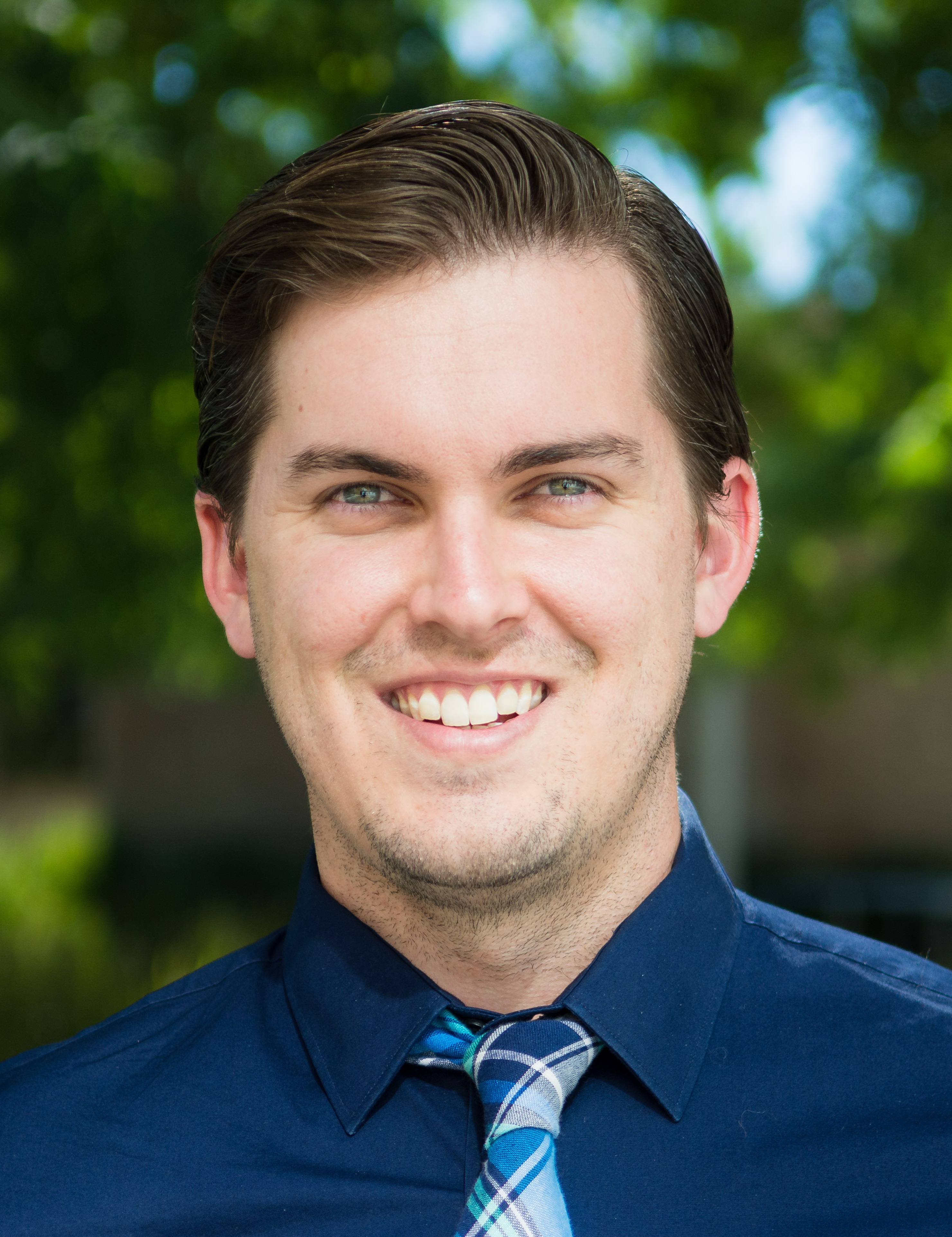 |
Differential Privacy, Presented by Matthew Graham, Center for Economic Studies, U.S. Census Bureau
(materials) (Juypiter notebook)
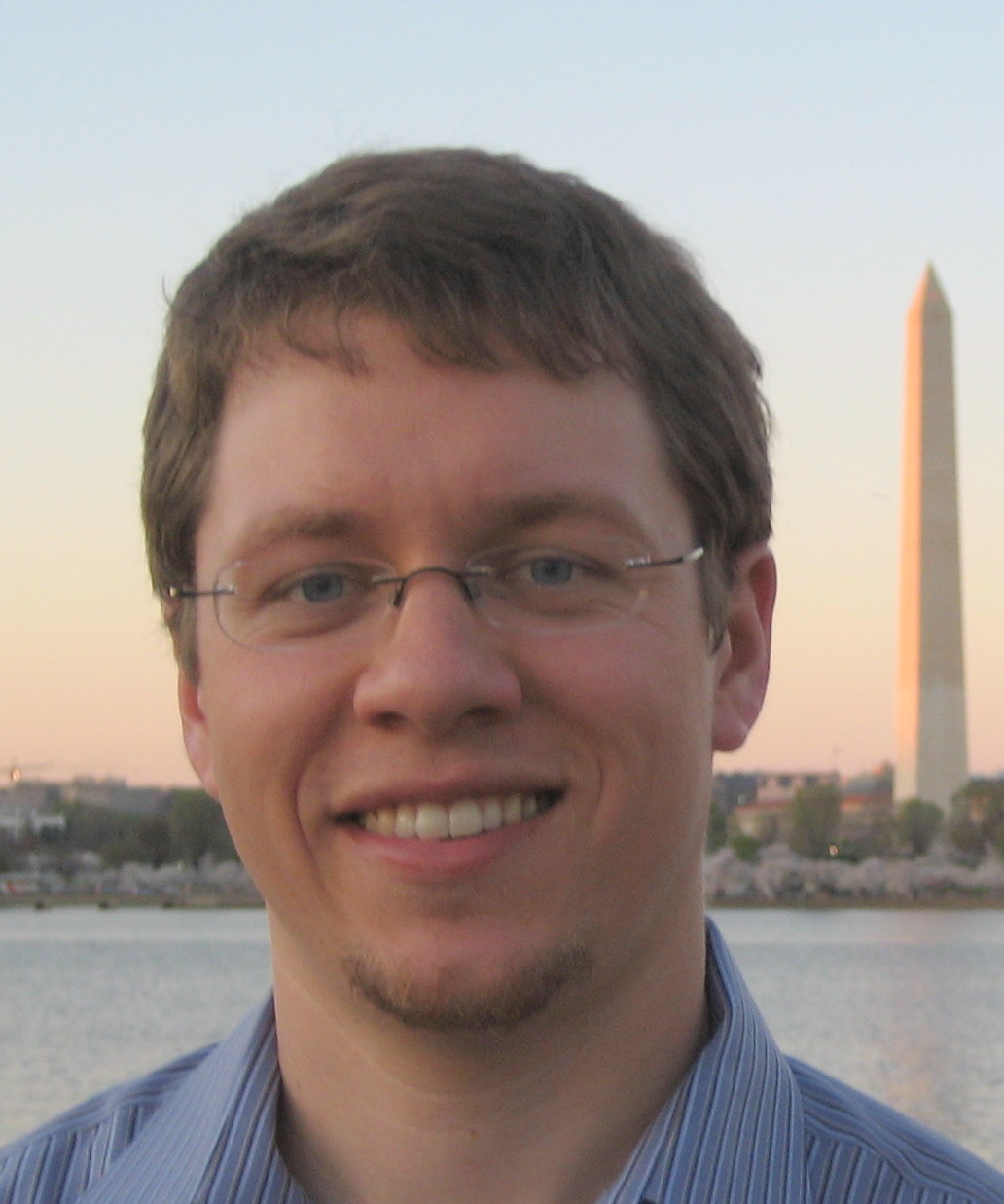 |
- Introduction to differential privacy methods, including key vocabulary (what is the privacy budget?)
- Examples from censuses
- Privacy considerations when using blended data
Bio: Matthew Graham is the Lead of the Development and Applications Innovation Group within the LEHD Program in the Center for Economic Studies at the U.S. Census Bureau. Over the last decade he has lead teams to develop and implement new confidentiality protection systems, new public-use datasets such as the LEHD Origin-Destination Employment Statistics (LODES) that use those privacy mechanisms, and web-based data dissemination/exploration tools such as OnTheMap, which was a winner in 2010 of U.S. Department of Commerce’s Gold Medal for Scientific/Engineering Achievement. Matthew has an MA in Urban Planning from UCLA as well as an MS in Mechanical Engineering and a BS in Physics from MIT. |